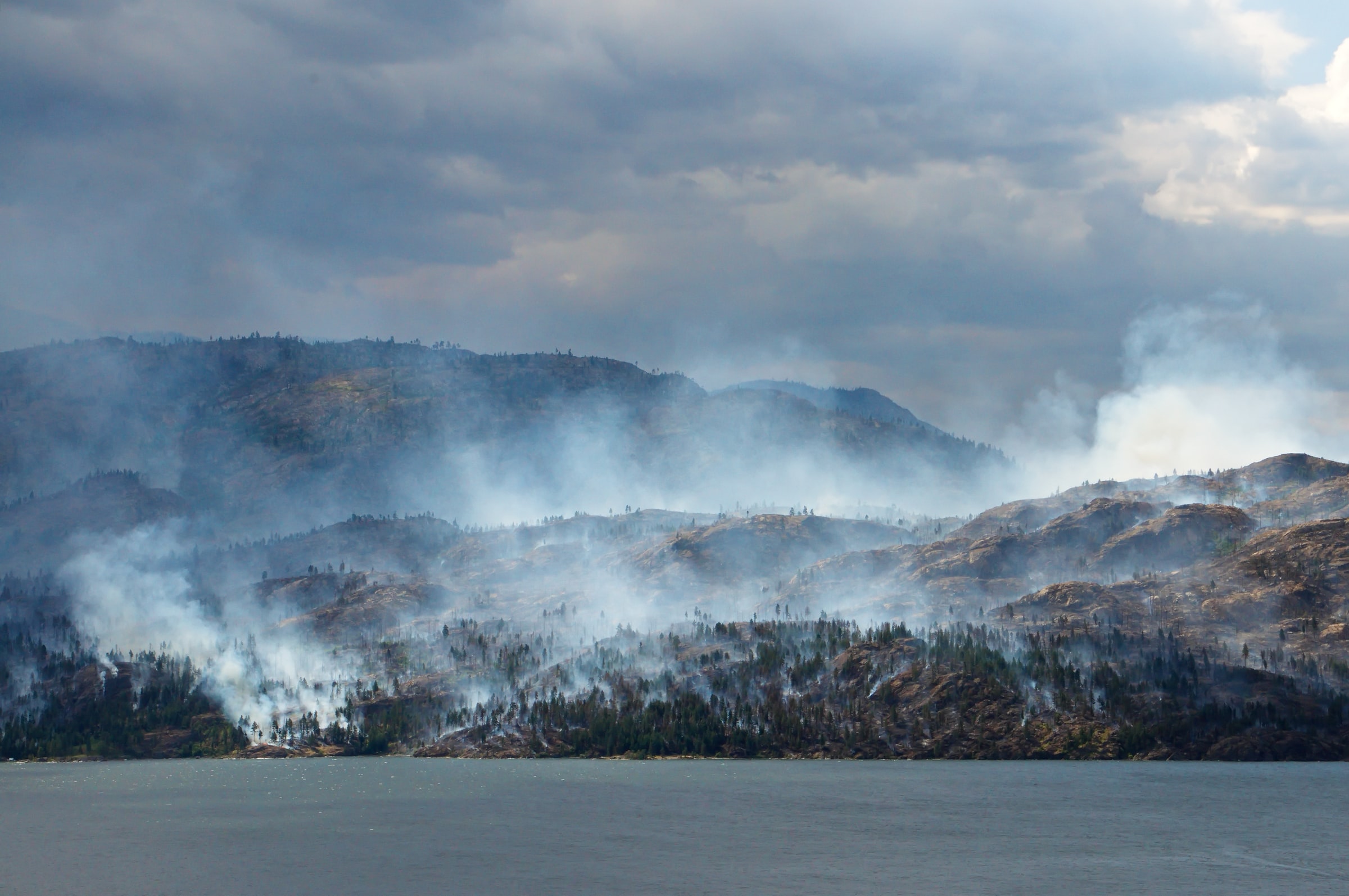
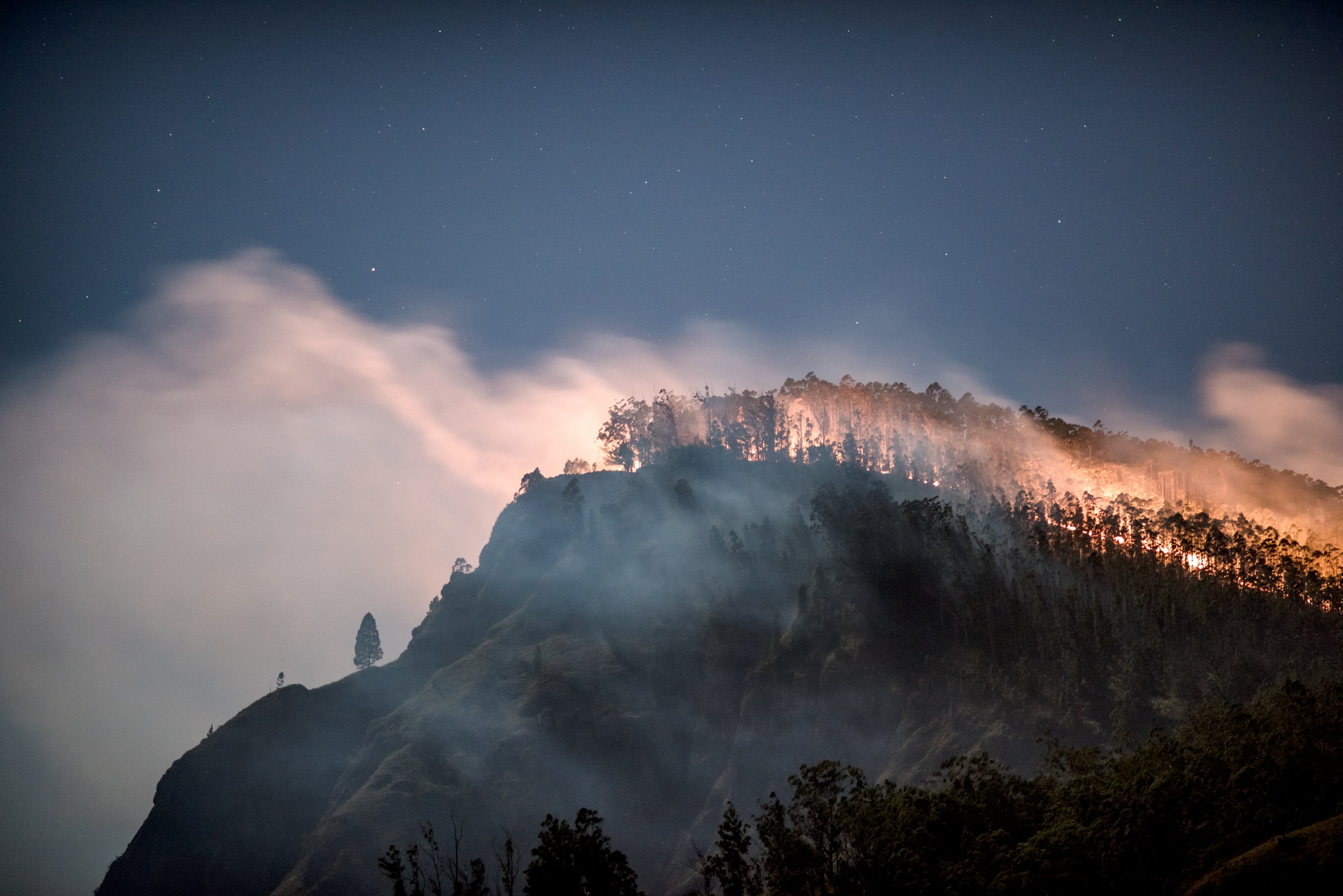
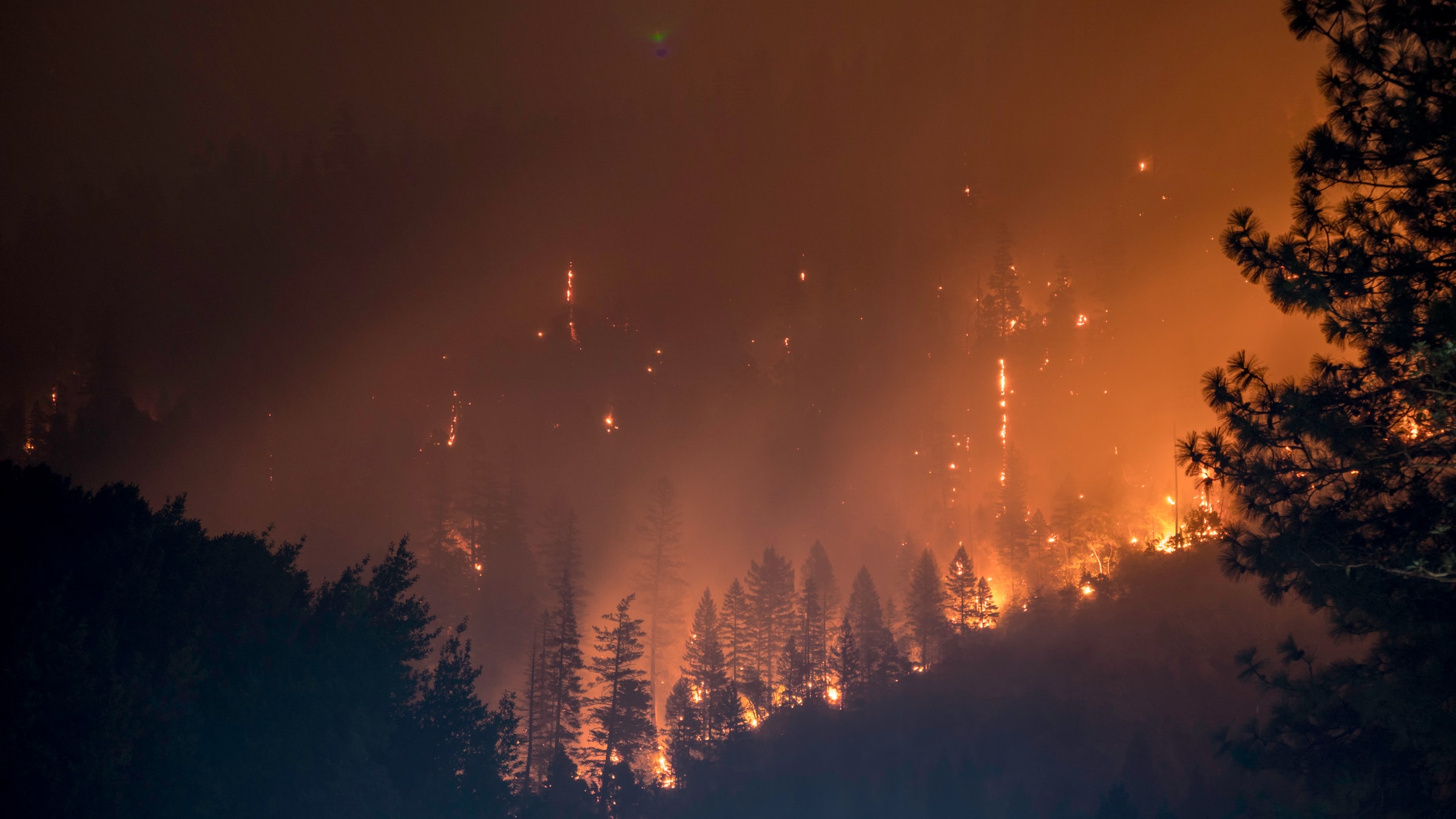
Work Package 1 – Coordination & Management
WP1 aims to ensure the successful implementation of the project in terms of time and budget. NOA is responsible for coordinating the activities, monitoring the implementation plan and data management, and reporting to ESA.
WP Leader: National Observatory of Athens (NOA)
Duration: March 2022 – March 2023
Work Package 2 – Big data acquisition and set-up of Data Cube
WP2 implements a Data Cube fit to the project needs. We will start from the definition of system requirements, specifications and data cube architecture, and then we will create labeled datasets with historical fires for the training and validation of our DL algorithms. Lastly, we will populate the Data Cube with space and non-space analysis ready data (ARD). We will develop scripts to automatically populate the data cube with satellite data, meteorological datasets from regional numerical weather prediction models, synoptic reanalyses, terrestrial ecosystem models, and in-situ observations. This WP is also responsible for the generation of terrestrial ecosystem model outputs.
WP Leader: Max Planck Institute for Biogeochemistry (MPG)
Duration: March 2022 – June 2022
Work Package 3 – Seasonal fire hazard prediction
WP3 aims at developing trustworthy data-driven deep learning architectures for seasonal fire hazard forecasting. As a first task, we will research multimodal EO data fusion techniques on top of data cubes. We will estimate diachronic trends in fused EO and non-space time-series of data related to forest fires and we will attempt to find teleconnection patterns between fire drivers at scales larger than Europe. Our second task is to develop a seasonal fire hazard forecasting system coupling DL and generic Terrestrial Ecosystem Models that estimate carbon and water fluxes, and stocks. We will analyse time-series of climate-related, vegetation and anthropogenic indicators regarded as fire drivers, vis-a-vis a database of burnt areas in Europe for the past 20 years. Subsequently, we will apply computer vision techniques to gain insights on how our forecasting model works. We aim to interpret and explain the DL data-driven model and identify causal relations between covariates.
WP Leader: National Observatory of Athens (NOA)
Duration: June 2022 – December 2022
Work Package 4 – Prototype system development
WP4 aims to develop a prototype production system. We will create fully automatic processing chains, from data access and downloading to remote sensing data pre-processing, AI inference and final product generation. We will also develop a web-GIS platform, i.e., a front-end application to disseminate fire hazard forecasts. We will design and implement visual analytics to assess fire risk on assets (population, businesses, infrastructure, agricultural production, etc.) and we will create interactive plots to assist model explainability and provide insights for causal relationships.
WP Leader: Harokopio University of Athens (HUA)
Duration: December 2022 – March 2023
Work Package 5 – Dissemination, Communication & User Engagement
WP5 aims to bring visibility to the project through scientific publications in peer reviewed journals and the design of communication material to reach different stakeholder groups. It involves the implementation of the project’s communication strategy, the creation of the website and social media accounts and the development of AI explainability animations.
WP Leader: National Observatory of Athens (NOA)
Duration: March 2022 – March 2023
Work Package 6 – Fine-grained Earth System Deep Learning
WP6 aims to expand on versatile transformers models, a type of deep learning architecture, to better capture non-local information from teleconnections (connections between distant climate phenomena) and the overall global state of the Earth. This includes analyzing time-series data to understand both local and global impacts and assessing how different temporal lengths affect model performance. The project also investigates the use of graph transformers to predict burned areas on a seasonal scale, exploring methods to incorporate global information and additional external biases to improve model accuracy. Overall, WP6 aims to advance the application of deep learning in understanding and predicting Earth system dynamics at a detailed level.
WP Leader: National and Technical University of Athens (NTUA)
Duration: February 2024 – September 2024
Work Package 7 – Visualizations toolkit
WP7 will deliver a visual analytics prototype, interactive, that will explore a selected set of observation- and model-based datasets for the interpretation and exploration of model results and the dissemination of project outputs. Standing on this perspective, WP8 will develop a data visualization toolkit with three main goals: (i)
the visualization of Earth observation data and outputs from SeasFire modeling approaches to support the
interpretation and the communication of project results; (ii) the interchangeable visualization of independent Earth observation data that is used as SeasFire modeling inputs; and (iii) Interactivity for analyzing the variable
importance in predicting burned areas.
WP Leader: Max Planck Institute for Biogeochemistry (MPG)
Duration: February 2024 – September 2024
Work Package 8 – Predicting CO2 emissions due to wildfires
WP8 aims to enhance the accuracy of estimating CO2 emissions from wildfires. By comparing existing carbon models with CAMS data and incorporating carbon flux data and Earth observations, the project seeks to refine estimations and mitigate biases. Current estimates rely on factors such as burned area, vegetation carbon stocks, and combustion completeness. While machine learning algorithms have been developed to forecast burned area patterns, estimates of vegetation carbon stocks and combustion completeness are model-based and utilize fixed emission factors. These methods, while practical for daily forecasting, may not capture larger changes in carbon stocks over longer time periods, especially for seasonal forecasting.
WP8 aims to address these limitations by:
(1) estimating emissions using the current carbon model used in SeasFire and comparing it to data from CAMS (Copernicus Atmosphere Monitoring Service); and
(2) exploring improvements over existing approaches by assimilating carbon flux data and integrating Earth observation-based information to refine model parameterizations and mitigate biases from model initialization.
WP Leader: National and Technical University of Athens (NTUA)
Duration: February 2024 – December 2024