Background
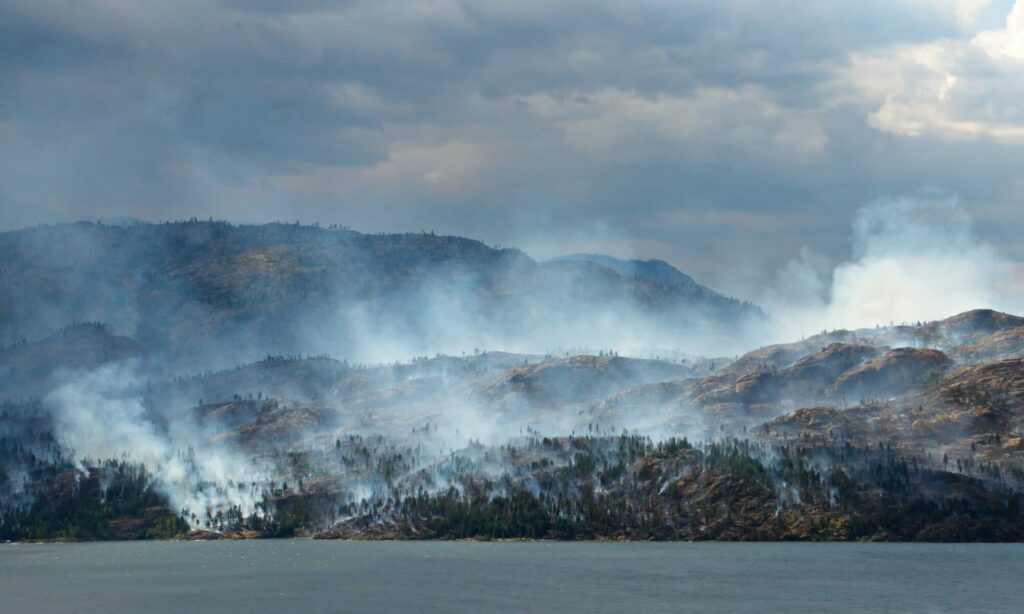
Fires are a major natural hazard, disrupting natural ecosystem services, causing loss of lives, properties, and infrastructure. We find it important to understand and model the phenomenon at a seasonal scale, as it is expected to become more frequent and devastating due to climate change. Studies have shown that fire does not behave the same way between different areas and years, which means that fire exhibits a spatio-temporal variability.
While working with short-term fire hazard forecasting in the DeepCube H2020 project (https://deepcube-h2020.eu/), we noticed that burned areas sizes in the Mediterranean area between 2009-2020 vary significantly between different years. Traditionally, this variability has been explained by simple statistical measurements such as the Fire Return Internal, or by using local variables derived from the main fire drivers, as input to tree-based Machine Learning (ML) models. Current approaches, however, ignore the fact that different areas of the world are essentially part of one spatio-temporally interlinked system, the Earth System.
The SeasFire approach
We propose to explore and capture these potential spatio-temporal asynchronous links happening between pre-occurring and non-overlapping atmospheric conditions and European fire regimes, to predict the seasonal burned areas sizes in Europe by leveraging two major advancements of our time: a) the availability of a huge amount of satellite data with a good spatio-temporal resolution, which will be used as fire drivers called the Earth system variables, and b) the progress in Deep Learning (DL) and especially Graph Neural Networks (GNNs) have proved capable of capturing the spatio-temporal interactions of the Earth System variables. We will develop a first of its kind prototype system that predicts the seasonal burned areas sizes for Europe, using global environmental variables, as well as terrestrial ecosystem modeling, and simulate their impact on local fire regimes.
Objectives
In SeasFire we aspire to answer three important scientific questions:
- What is the spatio-temporal contribution of the different fire drivers in the seasonal fire patterns in Europe and how do those fire drivers interact?
- How much do teleconnections enable us to anticipate seasonal fire patterns with high confidence in comparison to merely climate forecasting strategies?
- Can we use modern DL architectures to learn memory and lag effects in fire regimes and the possible teleconnections?
Project information
SeasFire is an ESA-funded project in the domain of EO science for society. Learn more: